The importance of goal-driven data
Luis Spencer Freitas, digital marketing manager for Pernod Ricard Americas, explores the current state of data gathering and analyses its true use in an ever-expanding digital culture
Anyone who works with digital mediums will always have some sort of fascination with metrics. One of the main pillars of sustainability for the advent of the digital age is the ability to measure and obtain data at a constant and up-to-date way that no other medium previously allowed. This allows for constant campaign optimisation and, of course, return on investment (ROI) evaluation. There’s nothing new with what I’ve just written – I’m basically stating the obvious.
Since metrics took an early stage in terms of value perception, most companies have been hoarding data in order to obtain the maximum amount of information possible from their campaigns – be it declarative or behavioural data. Every consumer input or interaction with brands is carefully being stored into vast databases that will then generate outputs, which brands can then use to optimise their communication or marketing efforts. This is the basic principle that originated what is today perceived as Big Data.
Big Data was born out of the constant data gathering that companies such as Google have had their business thrive upon. It’s such a huge amount of information that traditional data handlers or software cannot process. Therefore they require dedicated servers and specific mechanisms in order to extract some relevant information that brands can work on. As digital evolves more into a mindset and less as an isolated medium, the more we witness the data-hoarding trend. Be it through mobile devices such as smartphones or tablets, be it through desktops and laptops, or even through the emerging Connected TV technology, the fact is that every move the consumer makes is being tracked and stored in order for brands to build their relationship marketing strategies in more efficient ways.
This is all amazing in principle – but the fact is that most brands aren’t using their consumer data properly. In theory, having all this data should provide the necessary information to deliver true adaptive advertising and engage consumers at an entirely new level. But as studies show not only are brands not using their data properly, they aren’t able to define, at this moment, mechanisms to make this a cross-cultural tool in the company.
This is usually the point where you should take two or three steps back to gain perspective on this subject. The original hypothesis behind data collection is answering questions. What does my consumer want to see? What was the original objective behind getting his email and income? Why are we evaluating what sort of influence he has on his network? What is the purpose of identifying his favourite videos and channels on YouTube? The main role of data is to answer questions that are defined originally by the objectives we establish for a given task.
As data evolves and its gathering becomes easier, so does the temptation for creating huge amounts of useless (on a massification level) data that will only cloud brands from their purposes. These purposes and/or goals are becoming a post-campaign/action task, instead of starting points. Data evolved with digital, which means that the same fragmentation that brands have started to witness and act upon, concerning the different touch points, should be used in the same fashion when relating to analytics. This means that you should define the purpose of data you are gathering to begin with and not analyse everything just for the sake of analysing.
The exercise is quite simple – you have three defining levels of data objectives that play together:
Daily design news, reviews, how-tos and more, as picked by the editors.
Campaign-oriented data
Usually used to evaluate specific campaign objectives. As a basic example, if you are aiming at awareness, measure visits. If you want to evaluate content relevance, measure page views per visit. If you are evaluating content reach, evaluate shares and mentions. Loyalty, evaluate brand engagement on social tools (note – tools, not necessarily websites). Evaluate the consumer traffic from and out of the aimed medium, be it the Facebook page, the YouTube channel or the website. Create links with offline touch points that ascertain where is the consumer coming from or going to in order to evaluate medium efficiency. Define exactly what your objectives are and evaluate the metrics relevant to them, so you won’t become confused with data that does not answer your questions.
Relationship-oriented data
The hardest to define and to begin with. This is the data usually related to identifying consumer behavioural patterns usually used on CRM platforms. This means defining what are the consumer paths you want to track, what is the long term relationship objective and its quality on the several touch points, what is the engagement rate of the consumer with the brand on the several campaigns developed and, above all, what are the interactions obtained with the consumer in off-peak brand moments.
Channel-oriented data
Set apart what the behaviour expectation is from the consumer on a given channel. Here you can have two tiers:
- In terms of device-driven, if you are evaluating mobile data, consider that the input expected from the consumer isn’t the same as on a desktop environment. Mobile data comes from a more direct, primal impulse than a pondered, thought-based behaviour that is generated on an isolated medium such as the desktop or laptop computer. Also, the expected experience of the mobile environment is usually related to augmenting an offline experience or engaging in completely different ways. Definitely different from the more informative and extensive approach that the desktop experience brings you.
- In terms of context, you should also apply this to a social media environment versus a brand-owned website. If you are considering the relevance of your message on Twitter, you can probably evaluate follow-through leads into your other mediums, but stick to the objectives you first defined for your campaign on Twitter – is it growing your follower base? Is it engaging the right consumers, therefore obtaining a certain percentage of followers or retweets that fit into a specific age group or influencer profile? Choose exactly what your objective is on each device and follow the metrics that provide you the answer for it.
This article might lead you to some frustration, as you’d probably be expecting a detailed approach to which metrics are relevant for each objective and which aren’t. That’s enough material to write a book on. My objective is just to help you grow aware that Big Data is only as relevant as the answer it provides. Gather what is relevant and define the proper queries to obtain what you actually need from it. As digital evolved, so has the interpretation of the information you can obtain from it – as well as the segmentation and purity of that same data. Make the right questions to begin with and define your metrics. At the end of the day, that data will be a lot more useful than that enormous database you’ve been collecting for ages for which you don’t have the proper expertise to transform it into a value-added business inteligence.
Remember, it’s not about how much data you gather, it’s what you can achieve with it.
Thank you for reading 5 articles this month* Join now for unlimited access
Enjoy your first month for just £1 / $1 / €1
*Read 5 free articles per month without a subscription
Join now for unlimited access
Try first month for just £1 / $1 / €1
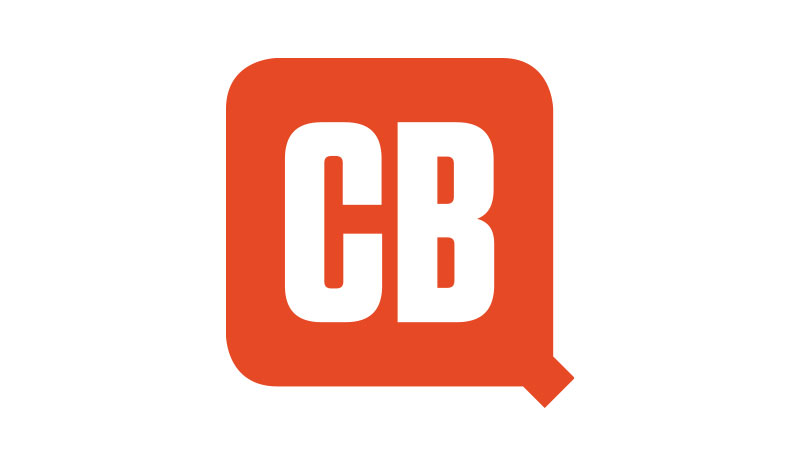
The Creative Bloq team is made up of a group of art and design enthusiasts, and has changed and evolved since Creative Bloq began back in 2012. The current website team consists of eight full-time members of staff: Editor Georgia Coggan, Deputy Editor Rosie Hilder, Ecommerce Editor Beren Neale, Senior News Editor Daniel Piper, Editor, Digital Art and 3D Ian Dean, Tech Reviews Editor Erlingur Einarsson, Ecommerce Writer Beth Nicholls and Staff Writer Natalie Fear, as well as a roster of freelancers from around the world. The ImagineFX magazine team also pitch in, ensuring that content from leading digital art publication ImagineFX is represented on Creative Bloq.